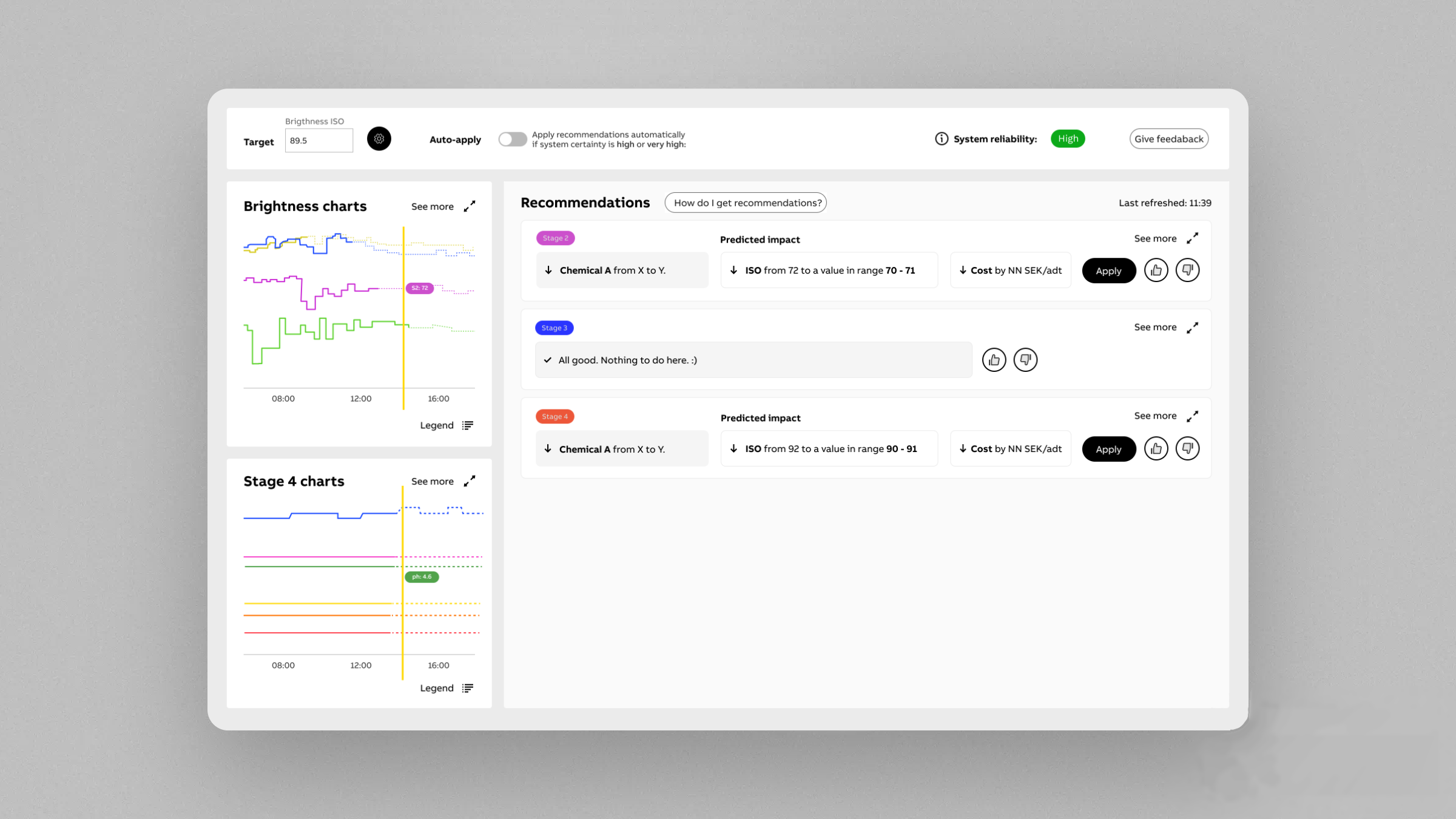

Explainable AI for the Process Industry | ABB
Process Operators in a Pulp and Paper Production Mill were provided with a recommendation system for optimising the use of chemicals in the process of bleaching pulp.
However, the adoption of the system was low. Most process operators have many years of experience and have a gut feeling about what they consider the best actions to take and find it difficult to trust a system if it does not always align with what they would do.
The use case provider was then in need of a design solution that could help increase adoption and provide a more transparent experience to process operators.
This work resulted in a research paper which you can read here.
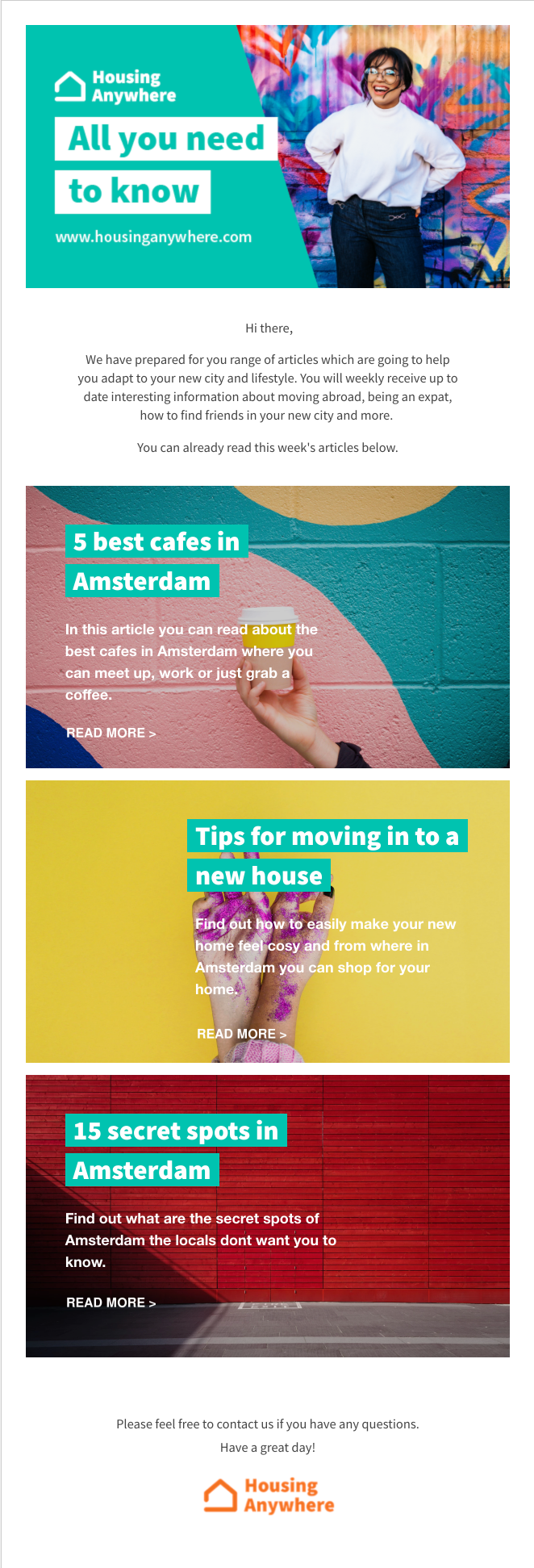
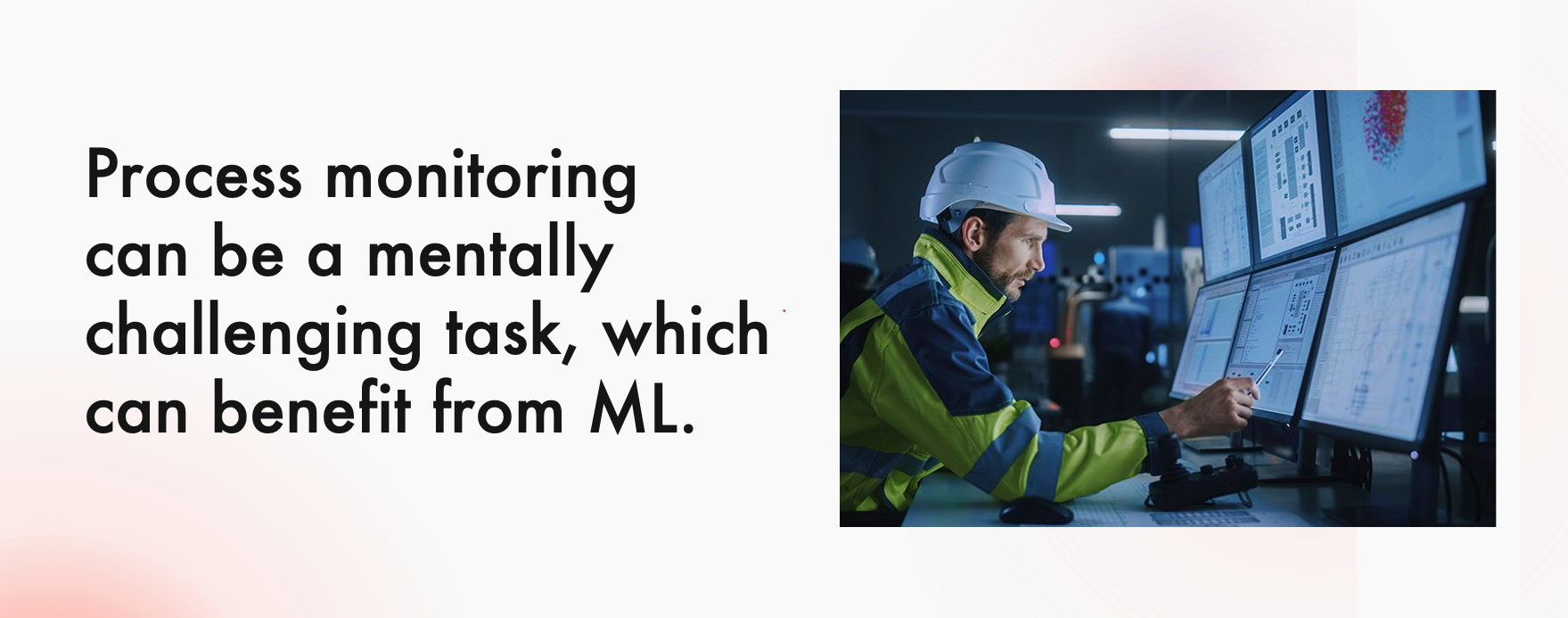
To uncover common requirements and explanation needs of experts in the process industry and design a better recommendation system for them.

For this project, the question-driven XAI methodology was used, which focused on discovering the questions users would want to ask an ML system and creating components that answer these questions.
To be able to answer these questions it was important to keep in mind who the user of the provided explanations is, because the way we explain should be different for different user types of smart systems.
Another aspect of explanations to consider is if the explanation is on a local or global level. Is it about the whole system or about a specific instance?
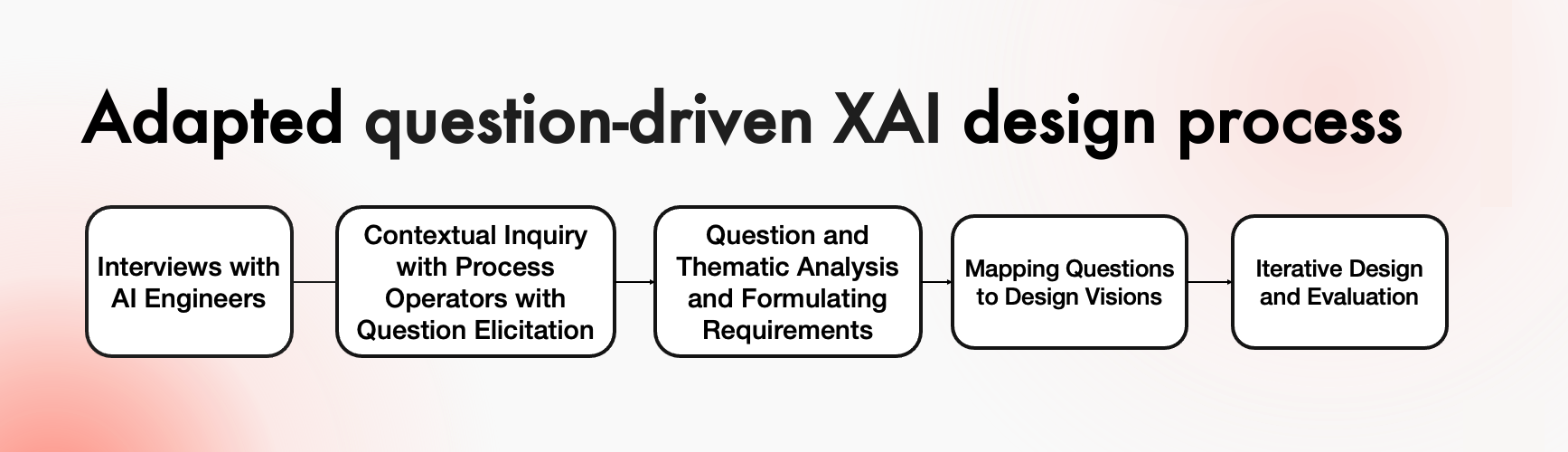
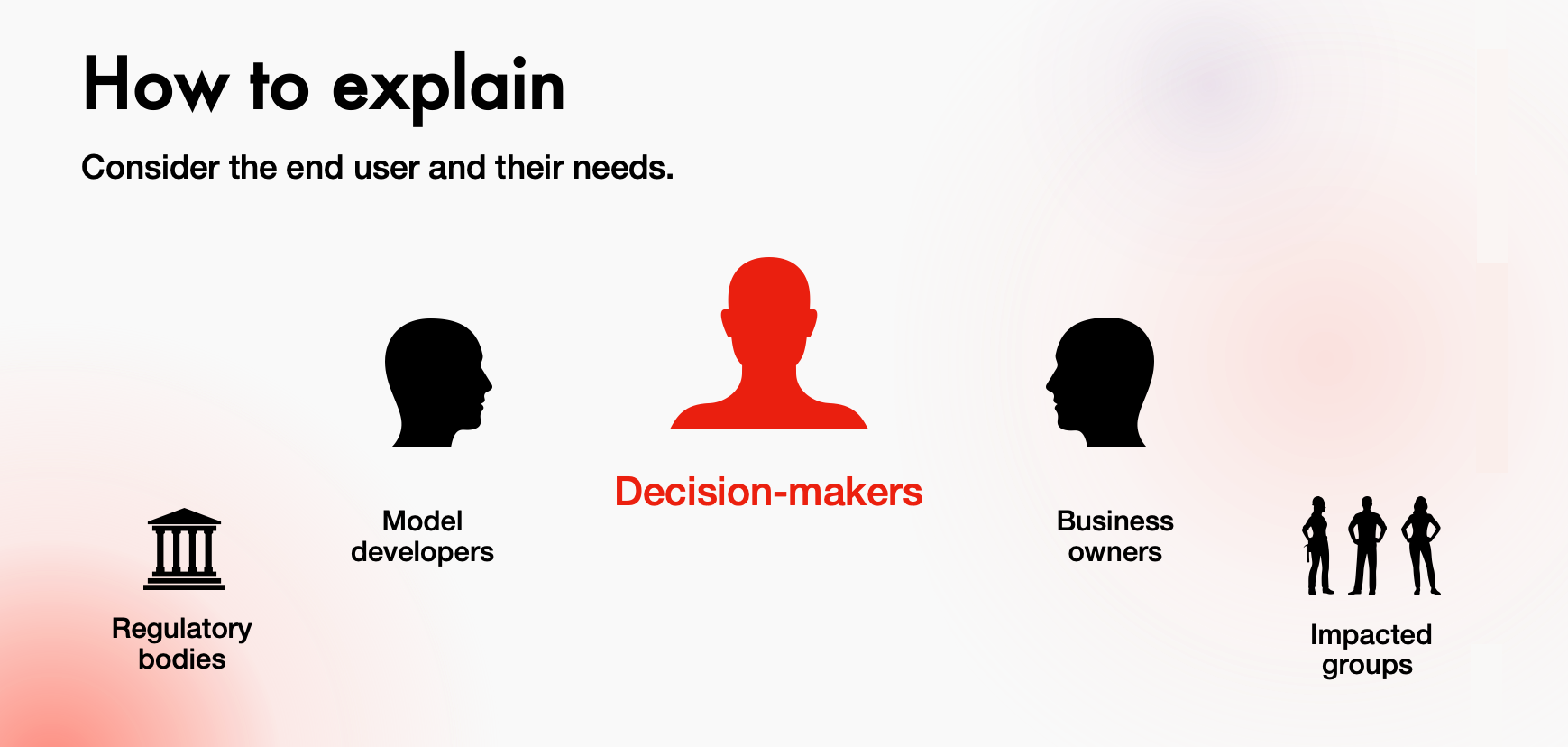
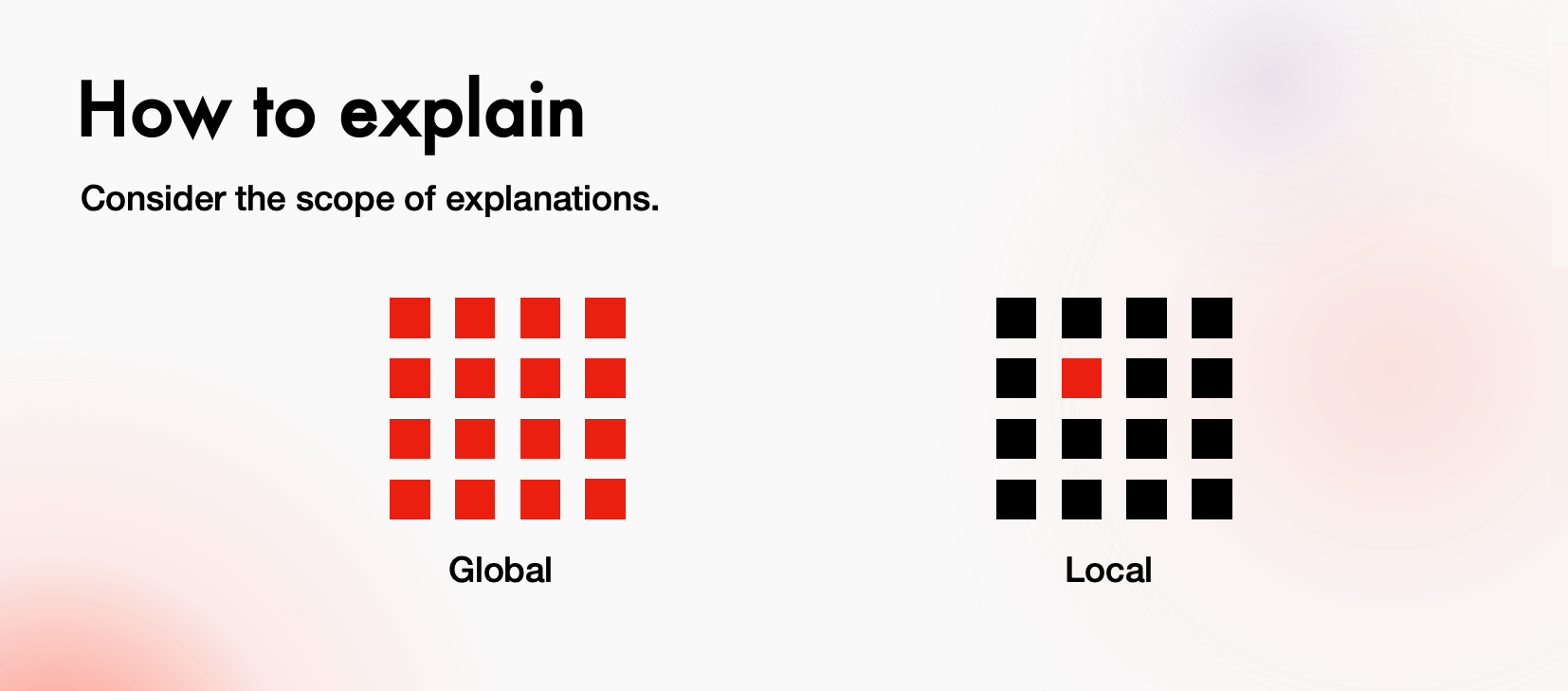
One useful way of differentiating between the different explanation needs is based on the types of questions users might want to ask the model.
In the domain of context-aware computing, the following types of questions are defined:
Input, Output, What, Why, Why Not, How, What If, How to, and Certainty


A field trip was organised where together with many of the partners of this EU funded projects we visited the paper mill. We observed the paper production process and afterwards interviewed process operators.
As there was already an existing system within this project we also talked to AI Engineers who worked on it to find out more about the way the algorithm works and how well its currently adopted.
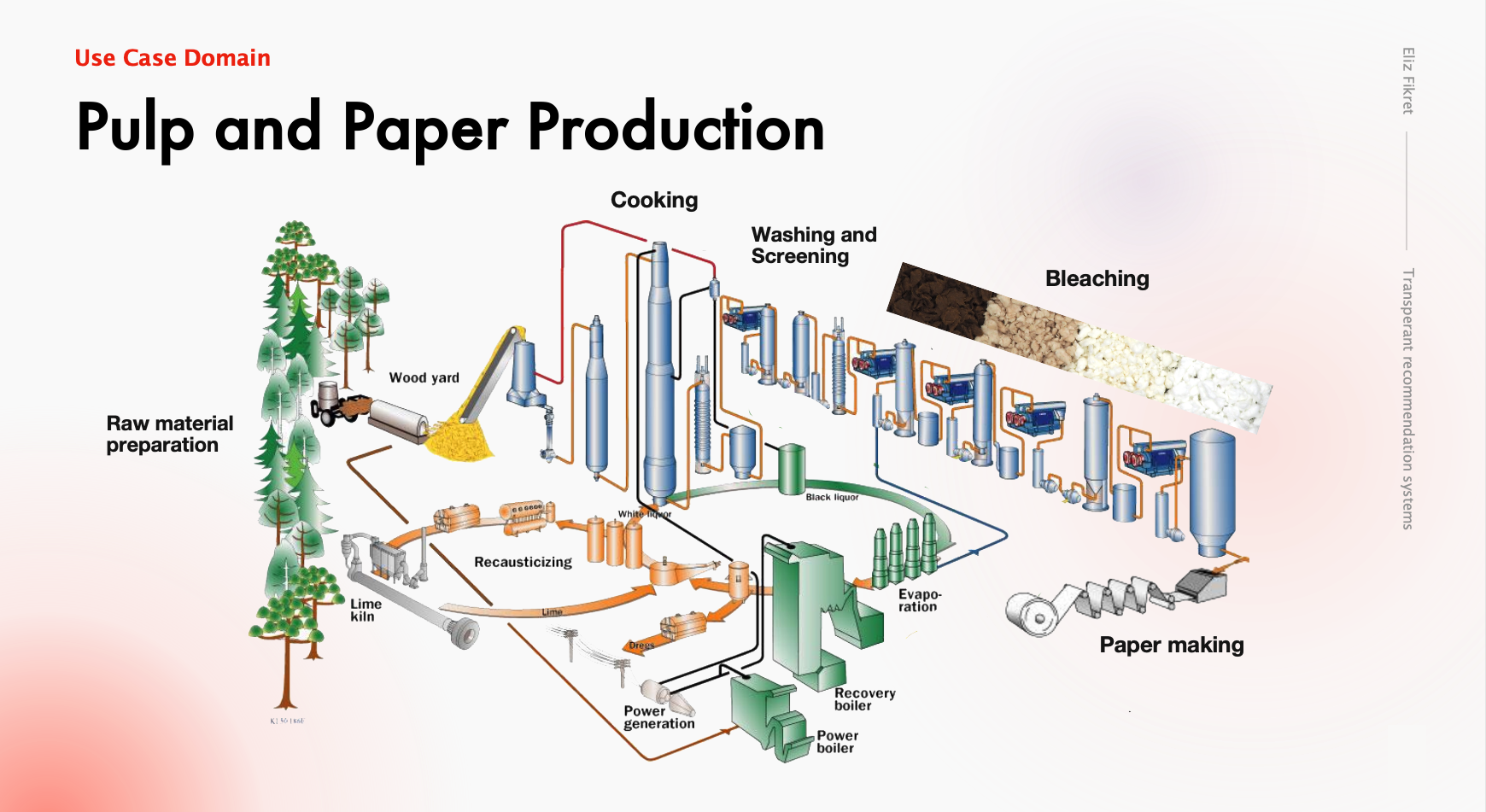
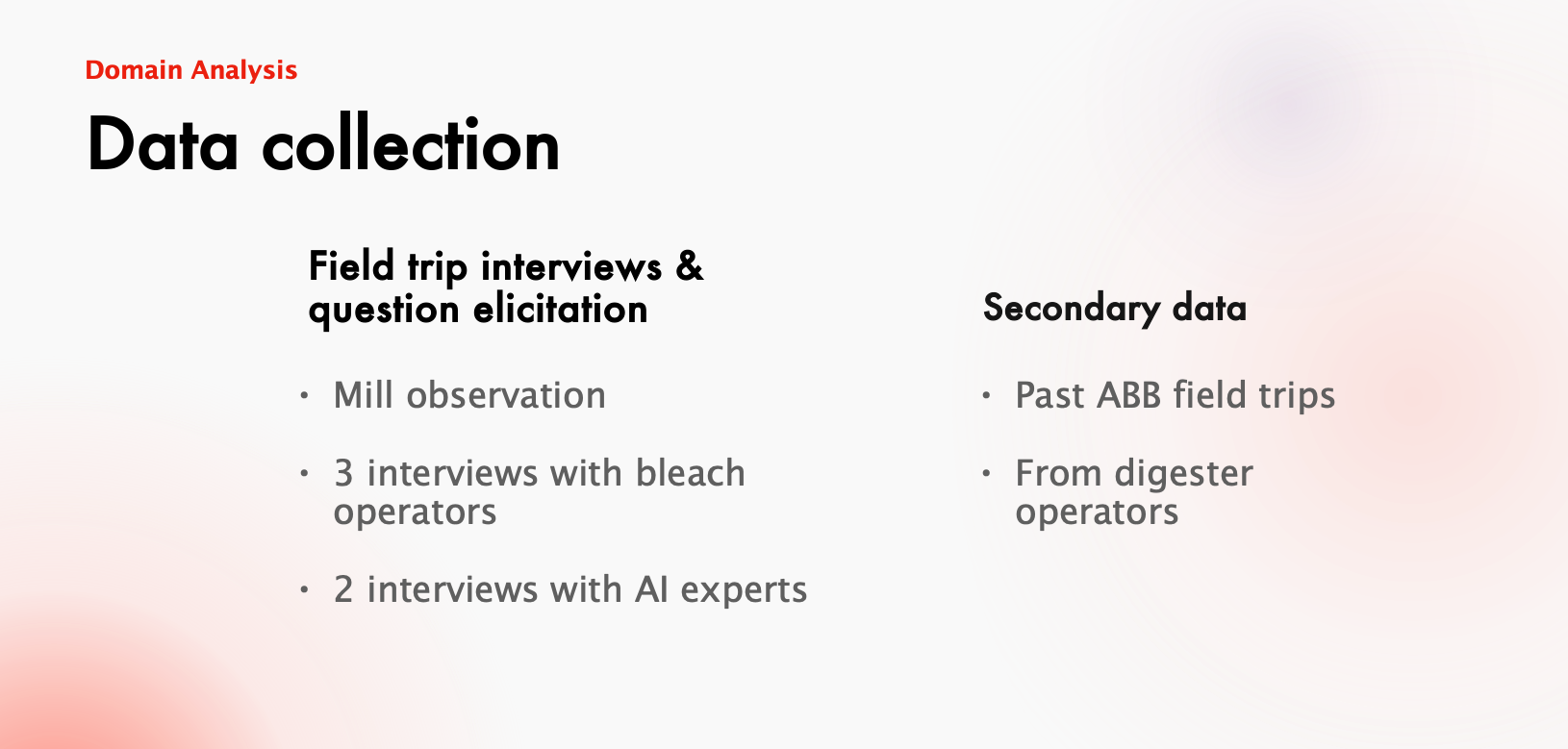
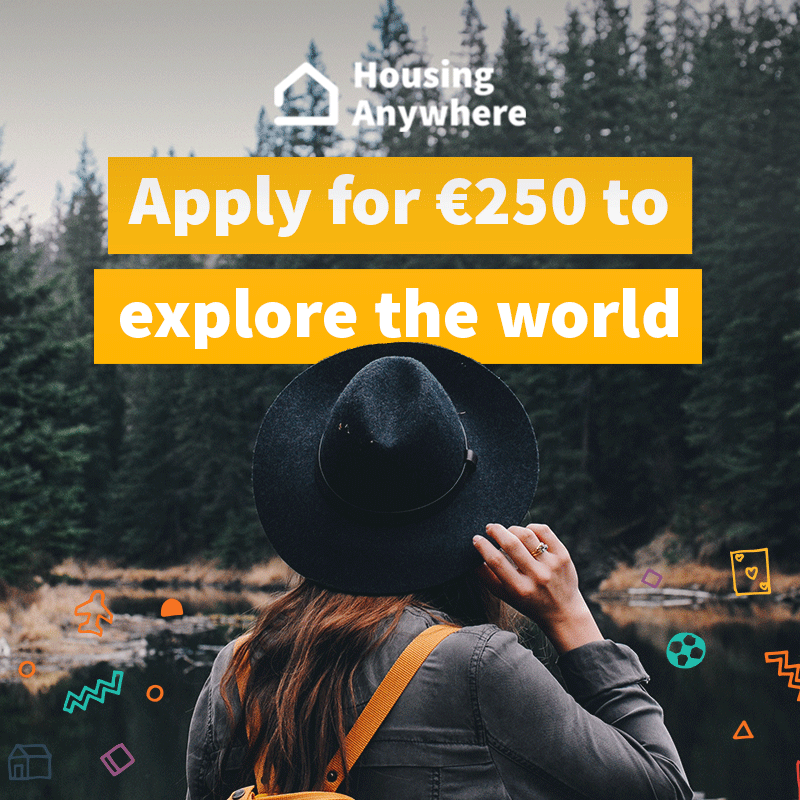
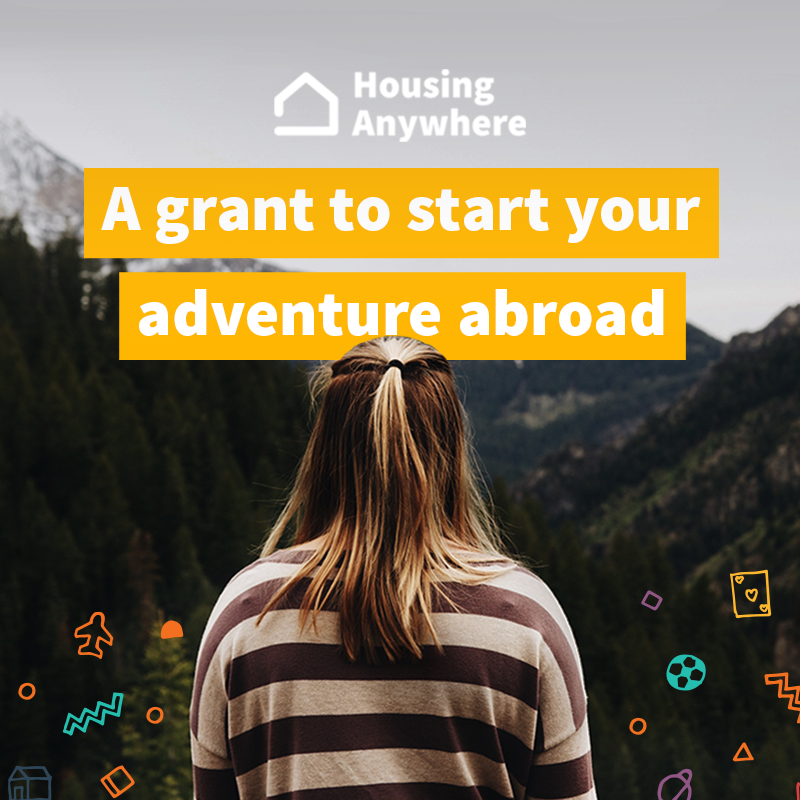
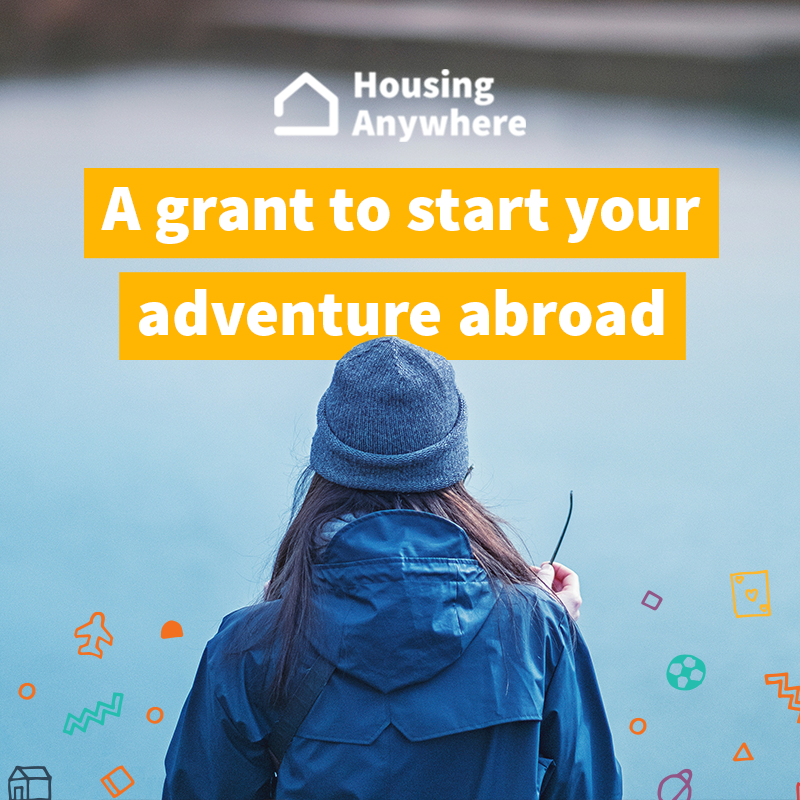
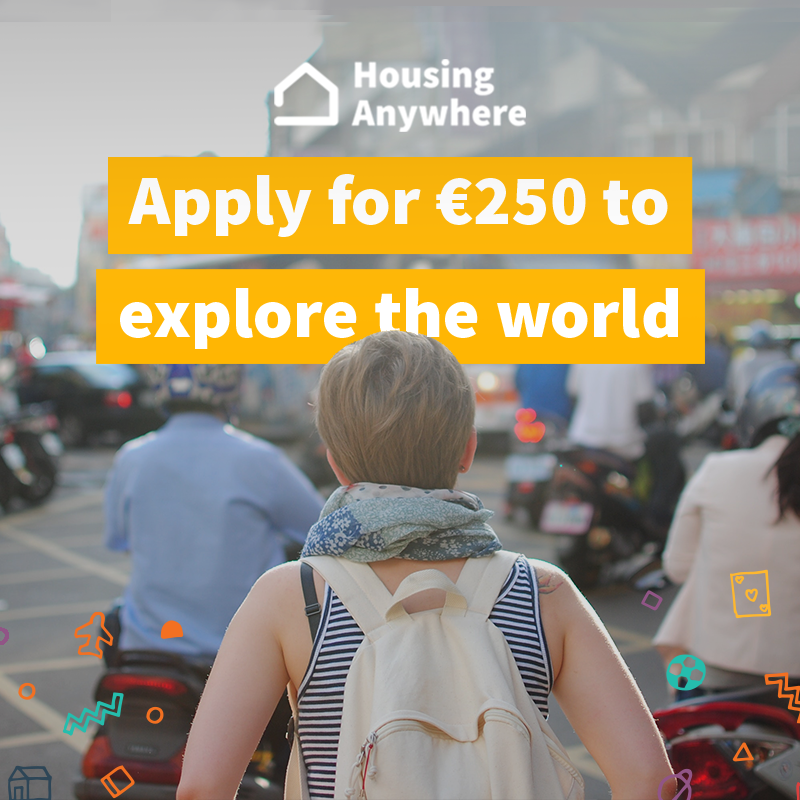
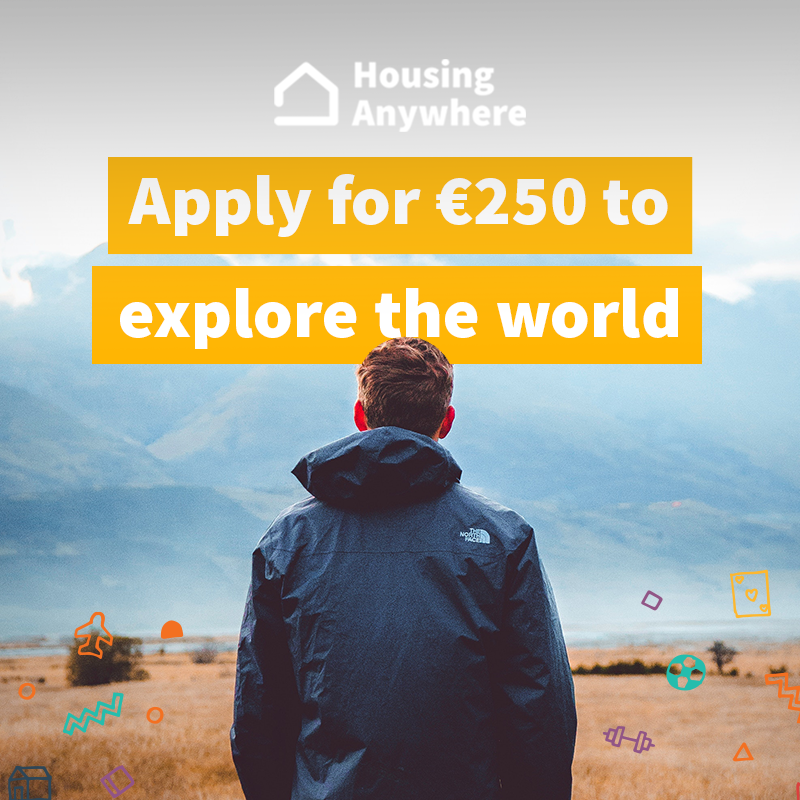
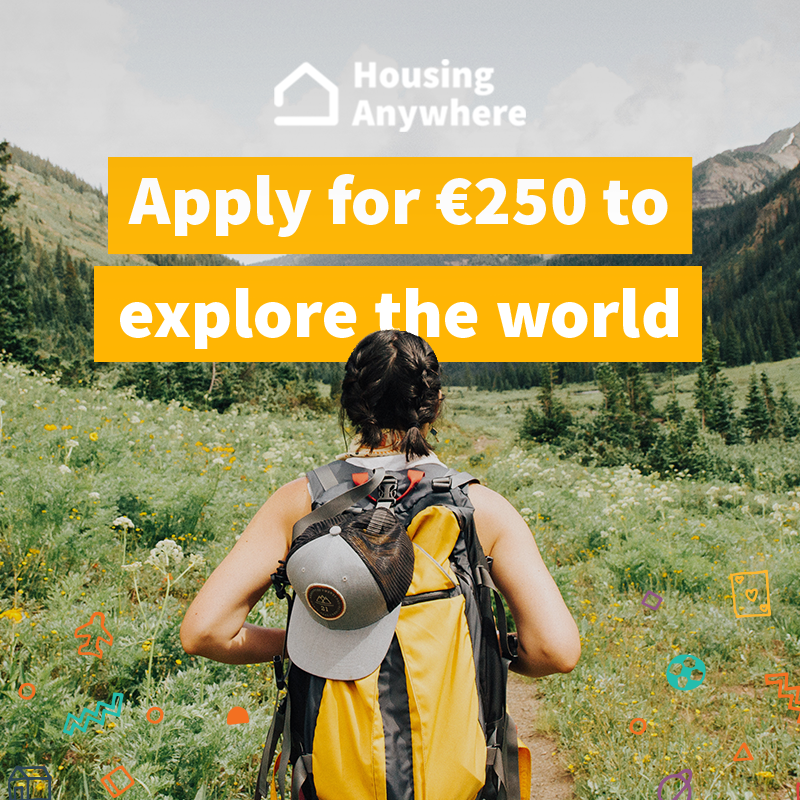
After analysing the data three scenarios were identified which you can read more about below.
Five different explanation types users were found to be interested in -Why, How, Impact. Certainty and What if. Impact was a new type I discovered which was not mentioned in the literature.
Moreover, the inductive thematic analysis reveled information about the operators' goals, needs and challenges.
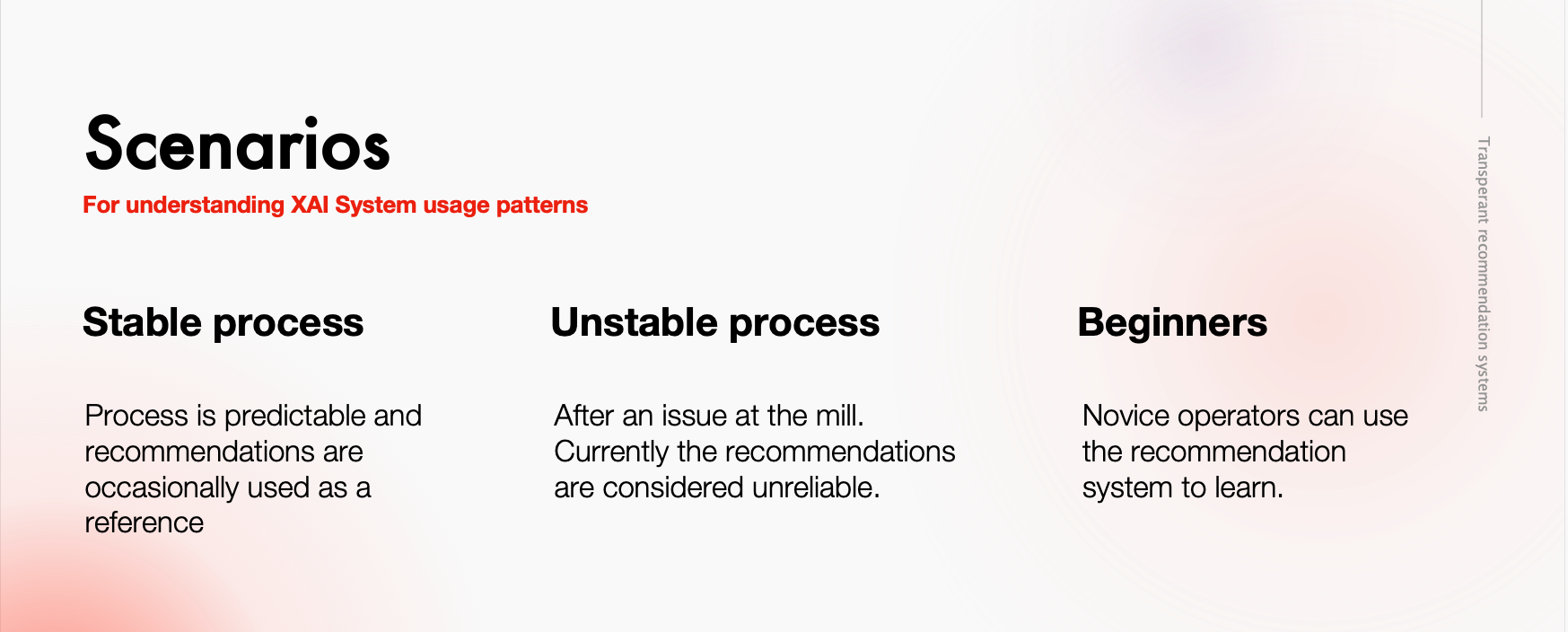
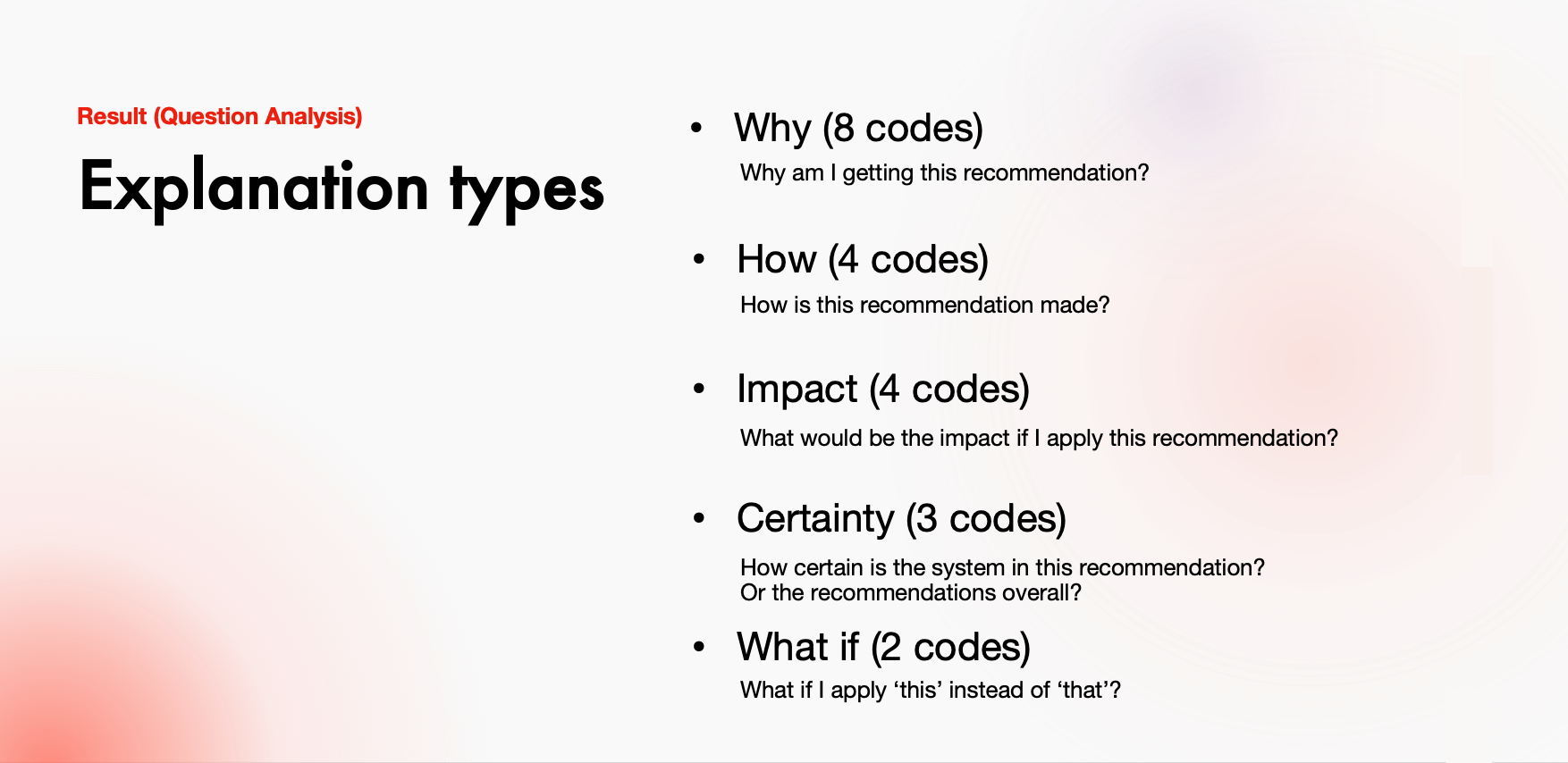
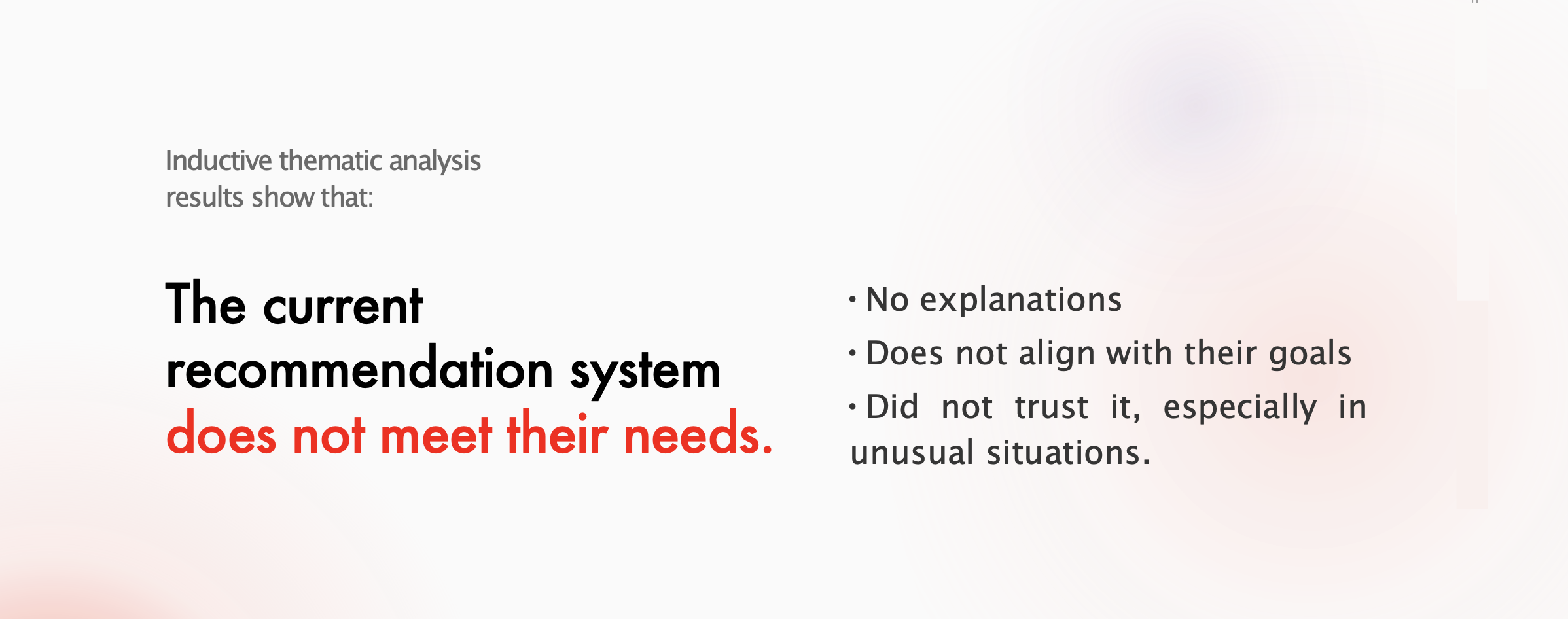
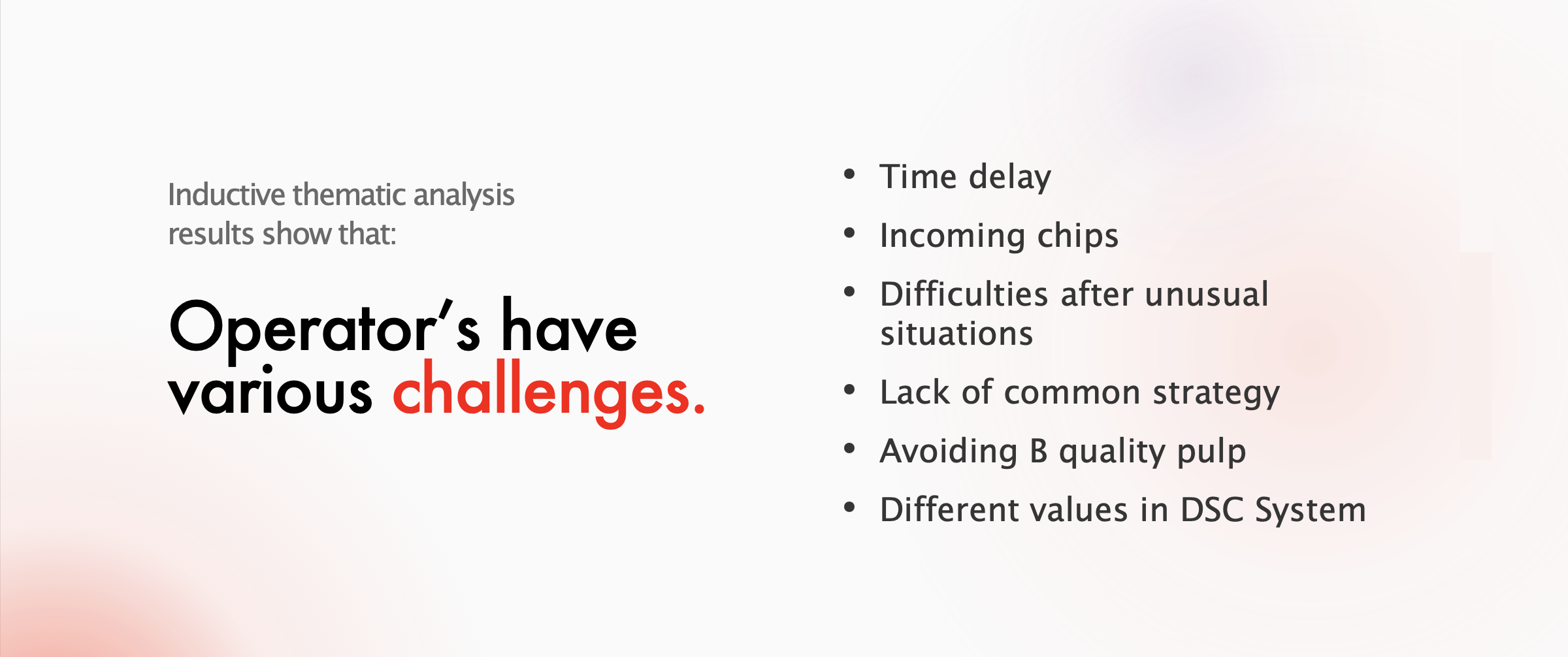
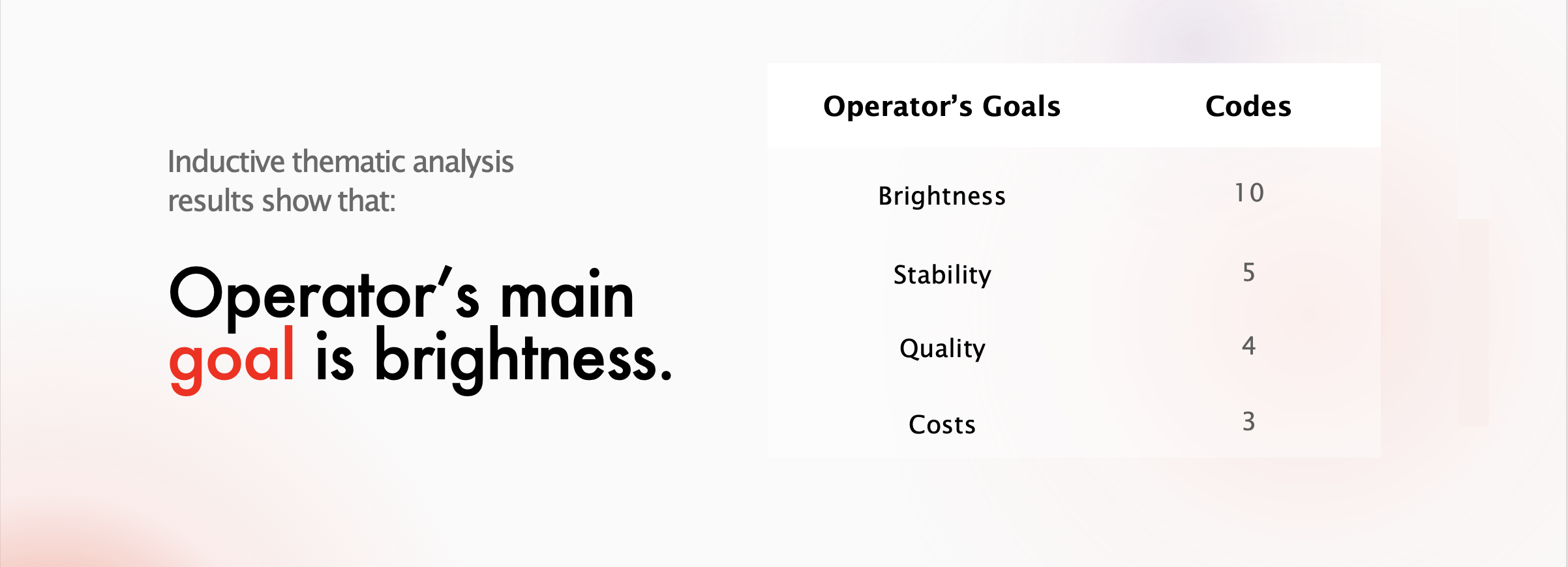
Nine user requirements were formulated based on the most commonly occurring codes within the thematic analysis and prioritised based on the goals of the user. These requirements were later considered in the design of the explainable AI system.

Illustrations of Rotterdam hotspots to be used in promotional materials about living in Rotterdam.
Tools:
Illustrator
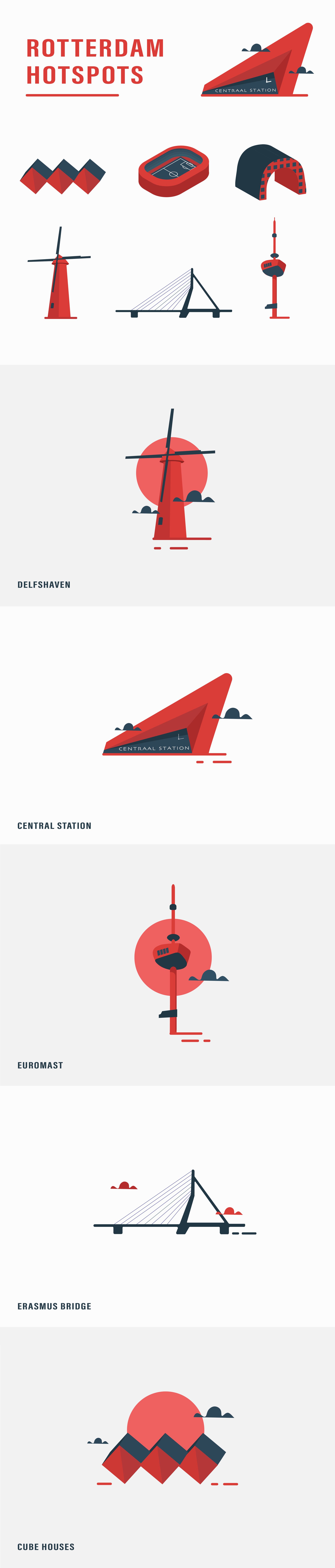


Based on the requirements stage and the explanation types identified in the previous chapter, an ideation workshop was designed. It was divided into two parts – ideation for a better recommendation system and better explainability.
A total of 5 how might we questions were asked during the session
• How might we give operators AI assistance that helps them achieve their goals?
• How might we give operators AI assistance that helps them during unstable situations like after a process stop?
• How might we explain why a user got a specific recommendation/prediction?
• How might we explain how the ML model 'thinks'?
• How might we explain a recommendation's/prediction's impact on other important values?
The questions that the XAI system needs to answer were mapped out to design visions based on the ideas in the Ideation session and ideas from literature. Then these design visions were sketched out.
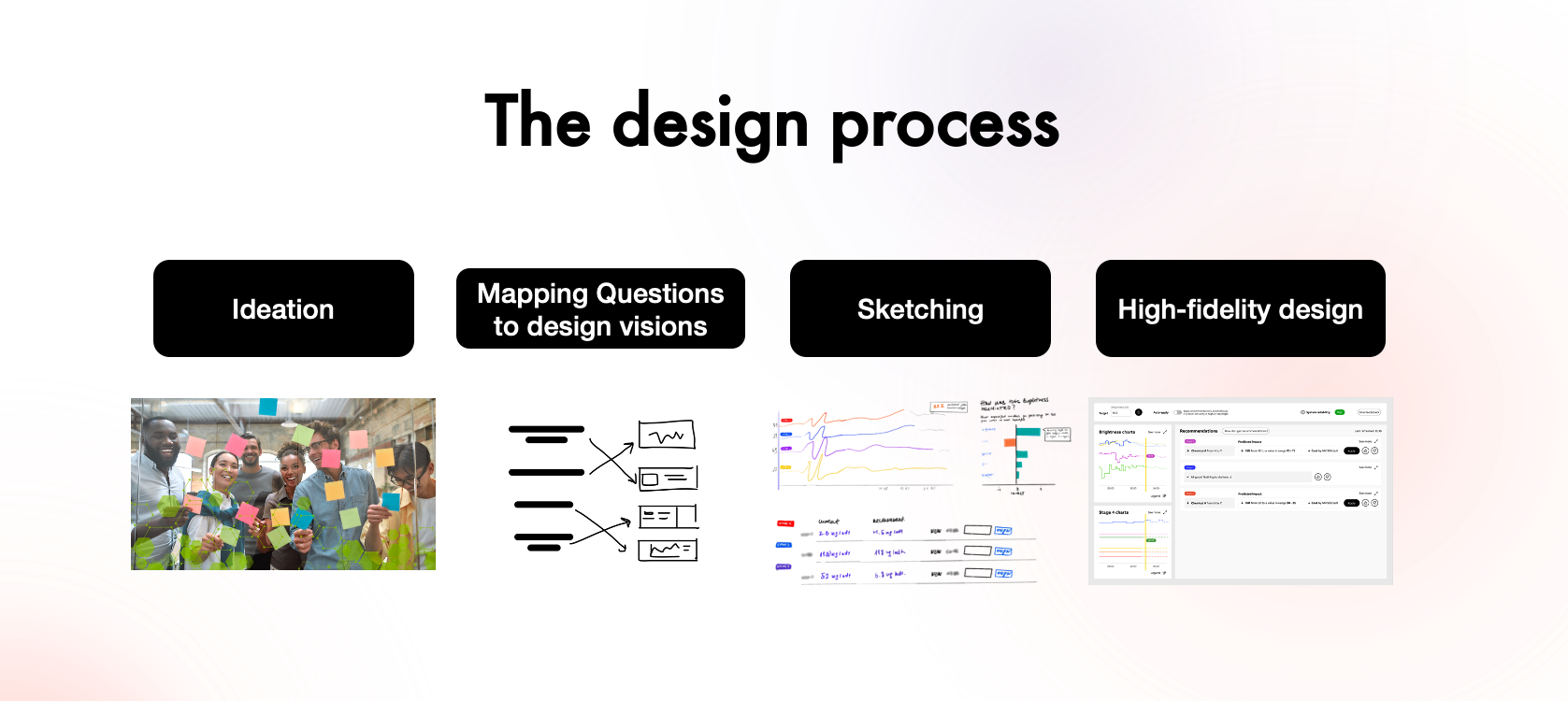
Low-fi prototype
Improvements
4 usability tests were conducted to evaluate the first prototype’s usability and clarity.
Afterwards, the prototype was improved based on the findings. Some of the issues spotted during the usability tests are described below together with their improvements.
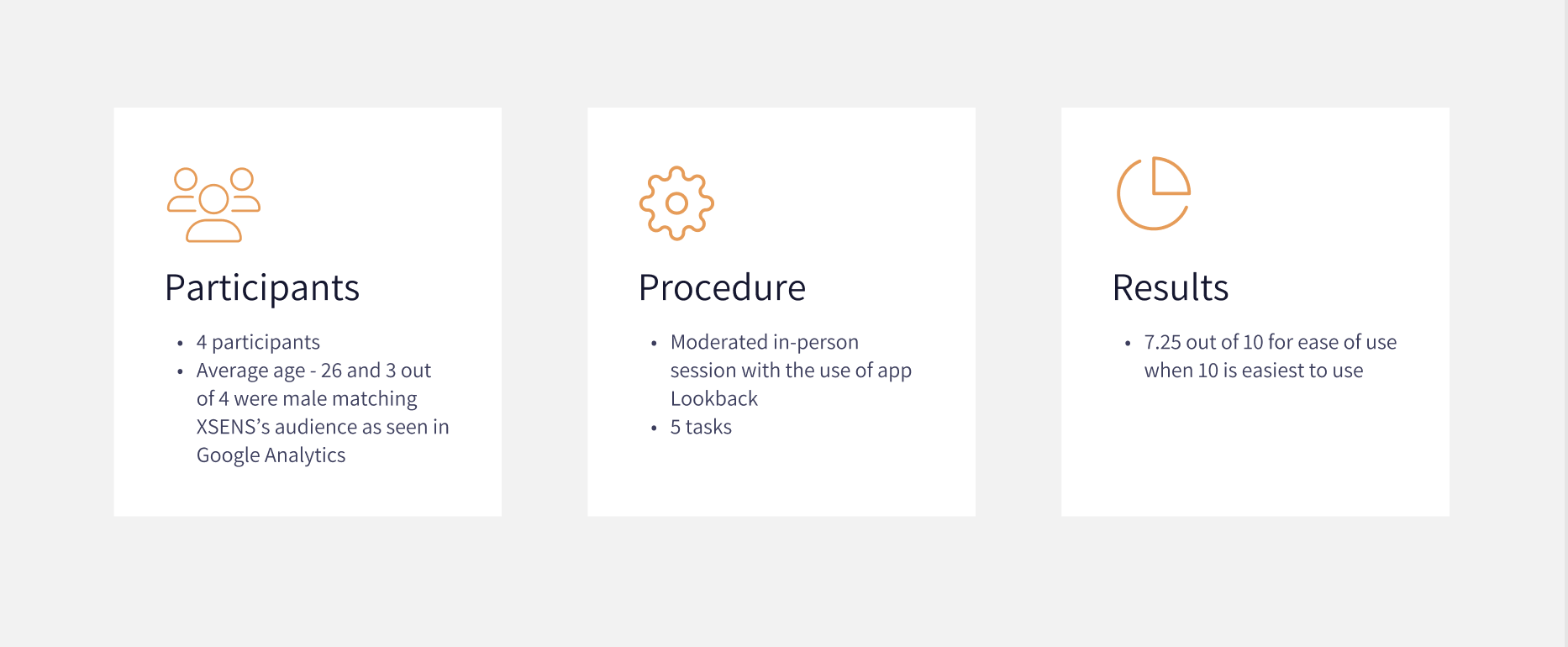
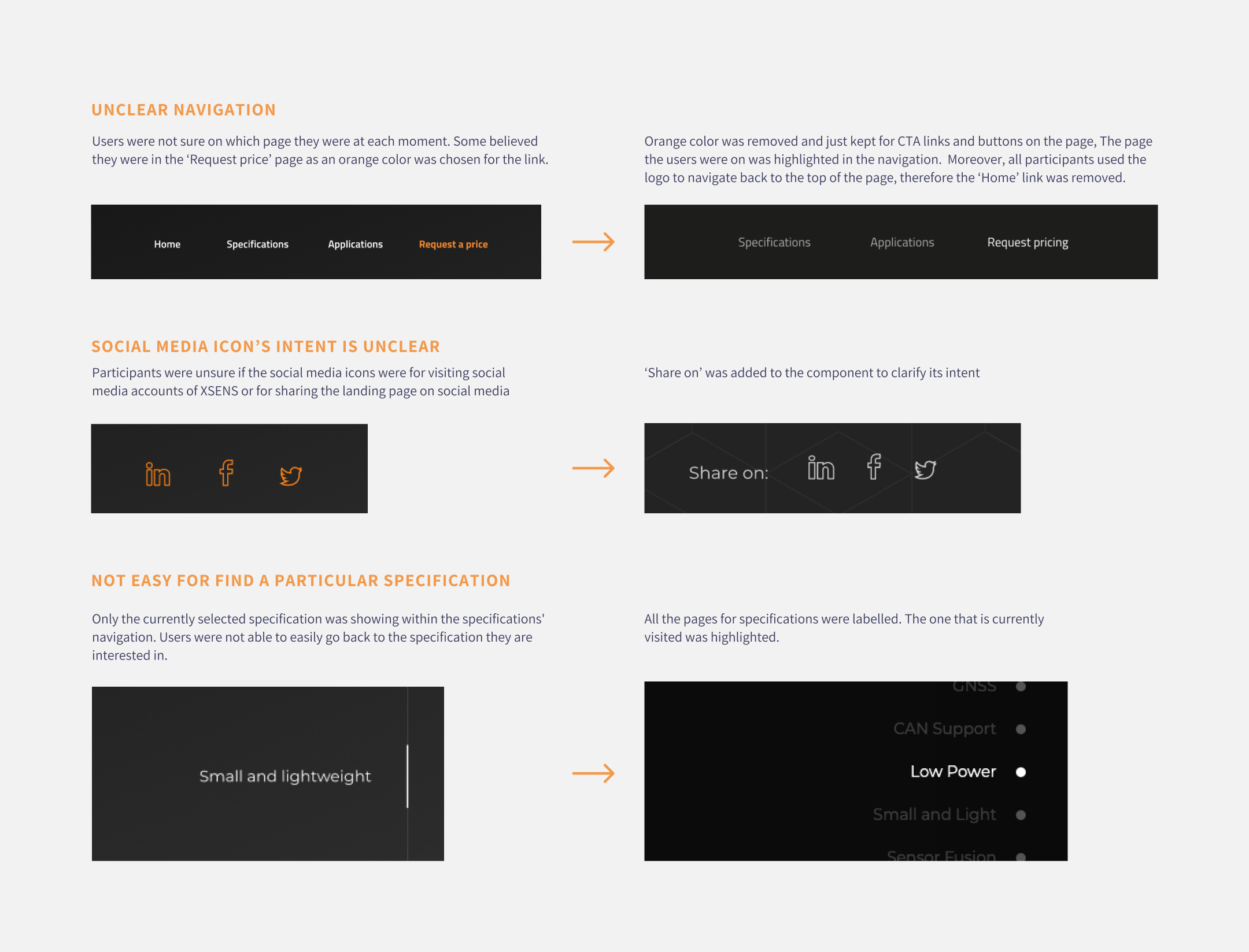
A simple design system was created to ensure consistency and speed up the high-fidelity design process.
You can access the design system from here.
.png)
.png)
.png)
.png)
.png)
.png)
Ideas from the ideation as well as proven data visualisation techniques (E.g. tornado diagrams to answer why questions) from the literature were used in order to answer the questions.
Two high-fidelity designs were created - one for the usual scenario where the system reliability is high and one for the unusual where something in the paper mill is going wrong.
You can interact with the prototypes at the following links or watch the videos.
Once the web page was live click map and heatmap analysis could be made as all animations were now running smoothly and all interactions worked as expected.
Tobii eye tracker was used in another set of user tests in order to be find out which elements on the page attract the most attention and which are missed and see if we could use this information to optimise the page further in the future. A heatmap was generated based on the results.
Moreover, a clickmap was extracted from Hotjar which showed us where do users clicked the most on the live page.
Usual scenario
Unsual scenario
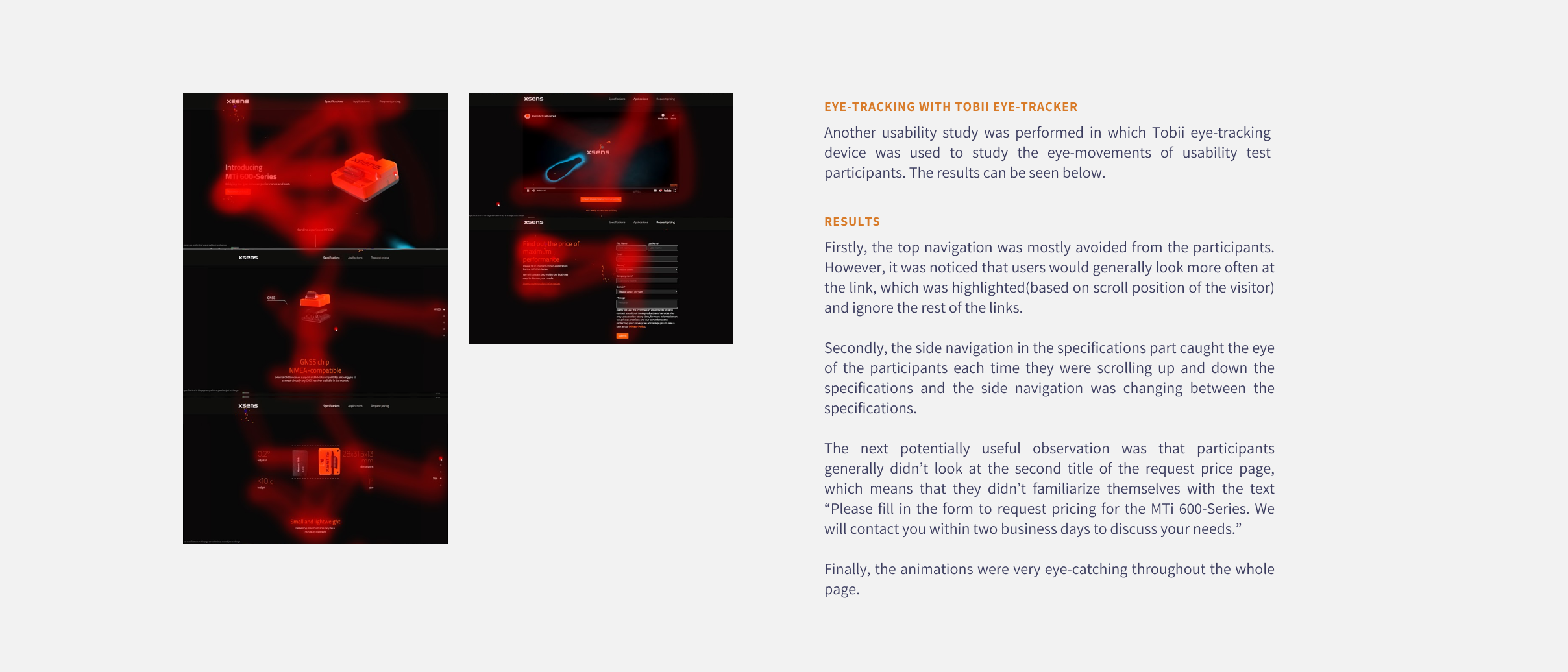

Three domain experts were invited for a prototype evaluation session. The session included a prototype walkthrough and a discussion. The goal was not to test the prototype's usability, but the design visions inside of it and if they help produce a more transparent experience for users.
Moreover, as this project was part of a European research initiative, another aim was to formulate recommendations for UX researchers working in process domains. You can read the recommendations below.
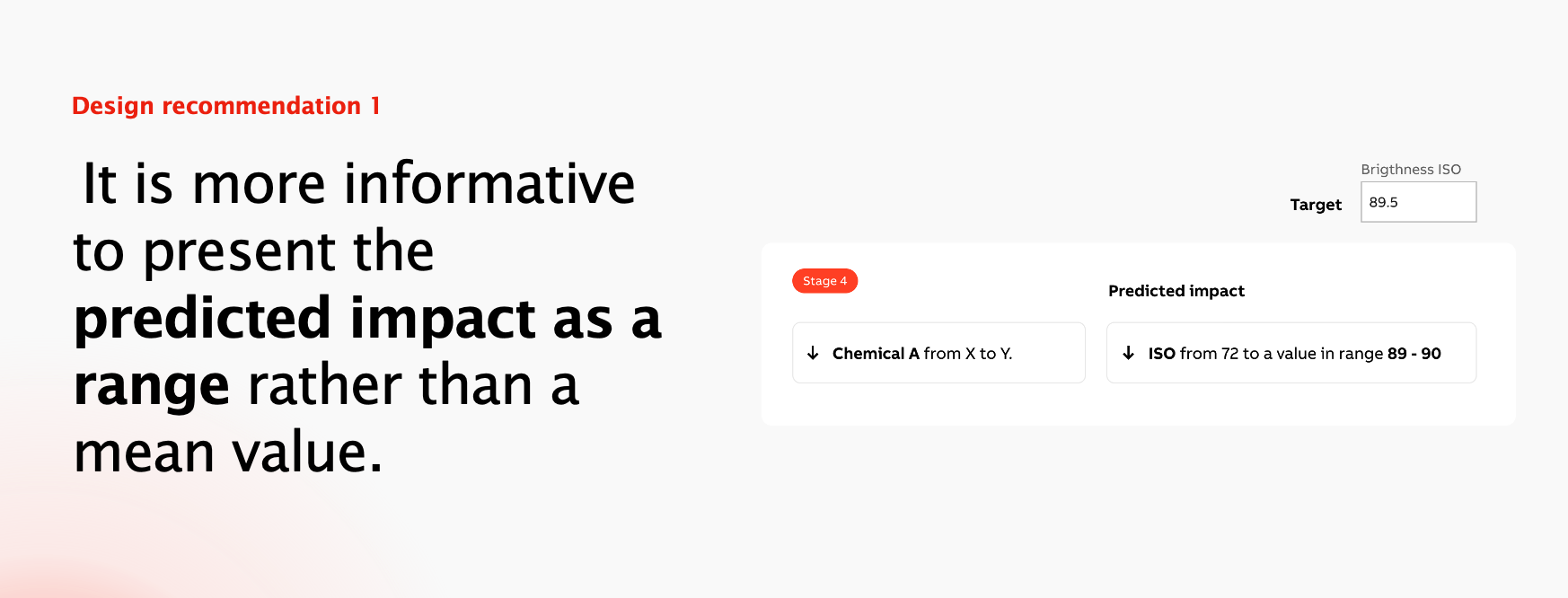
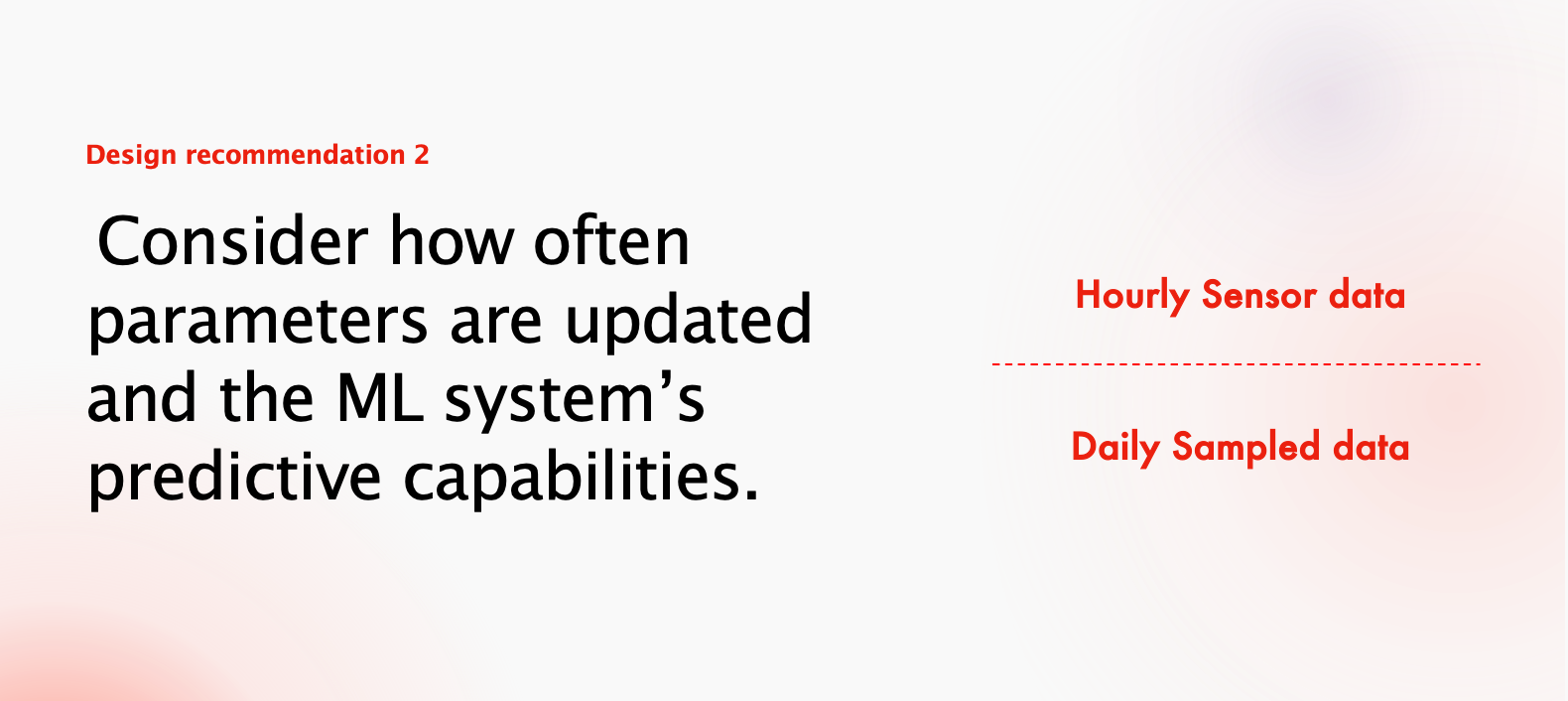

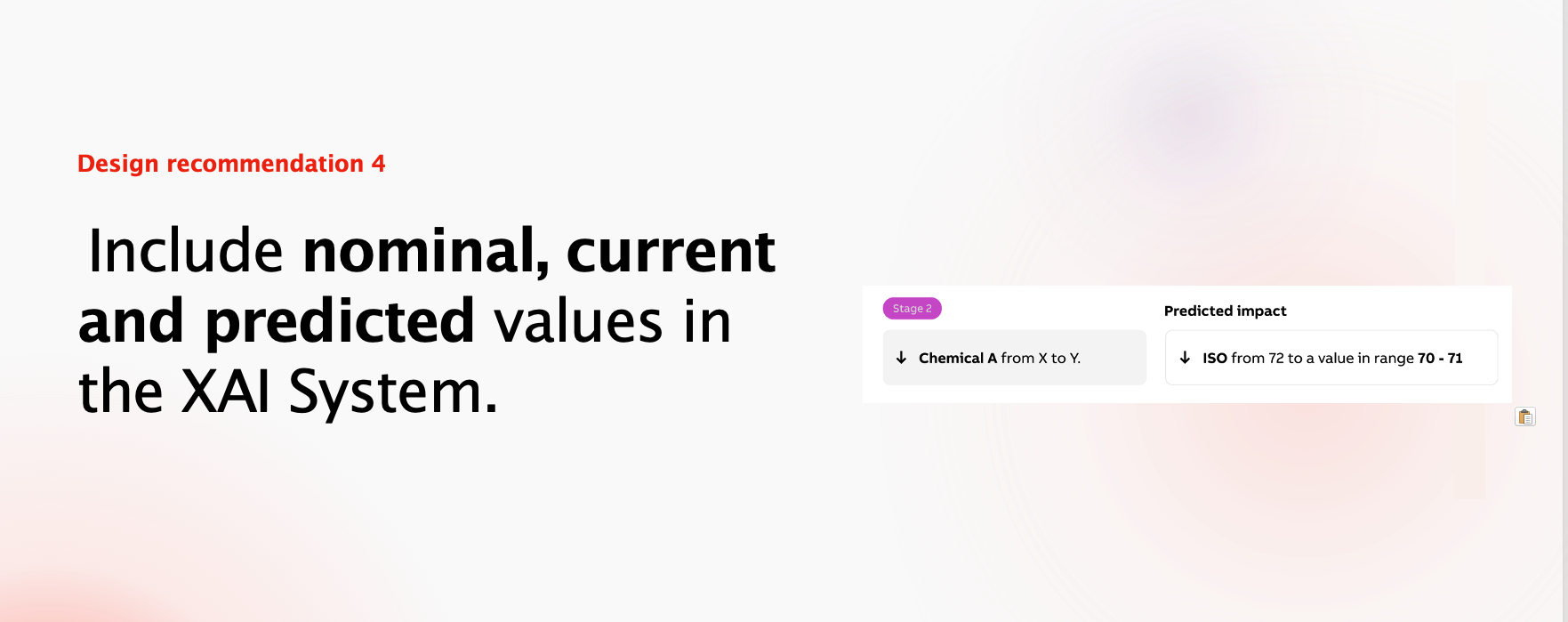

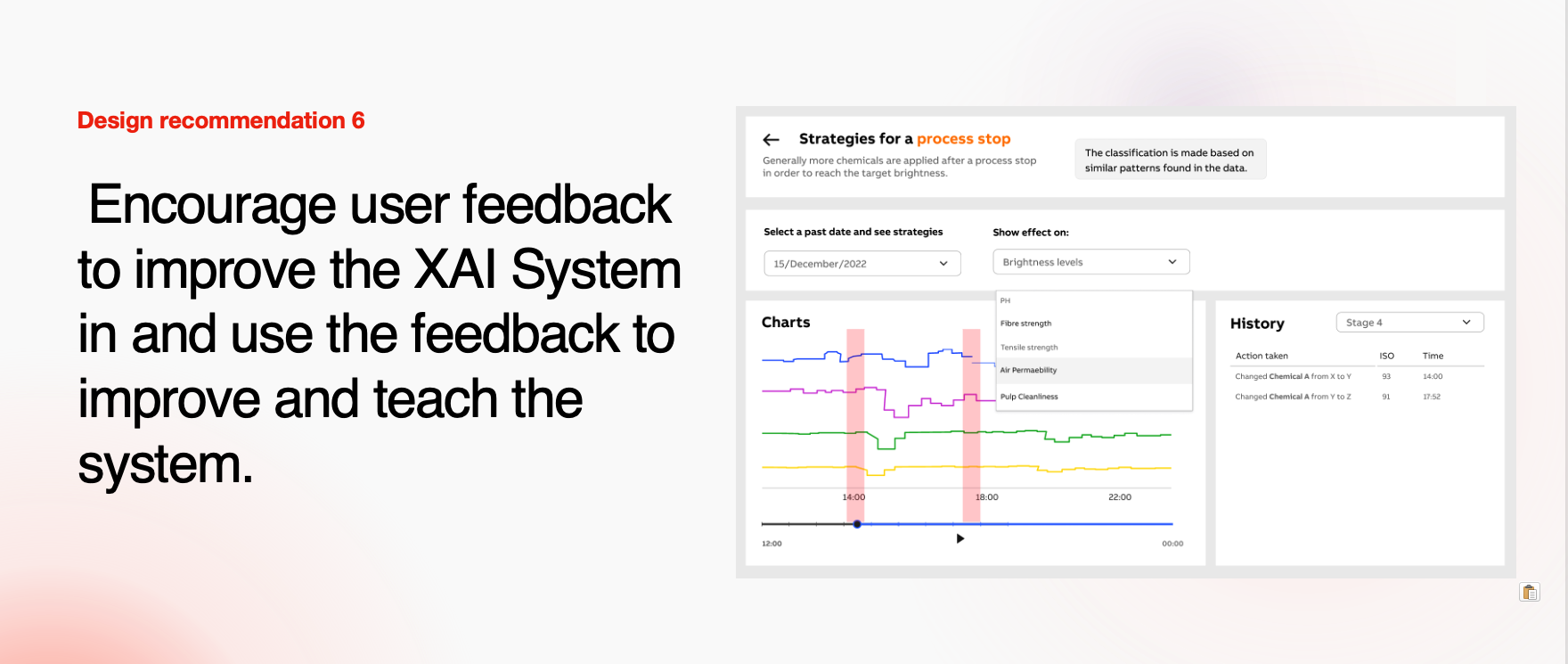
Improvements were made based on the major issues found during the usability tests. Some are mentioned below.
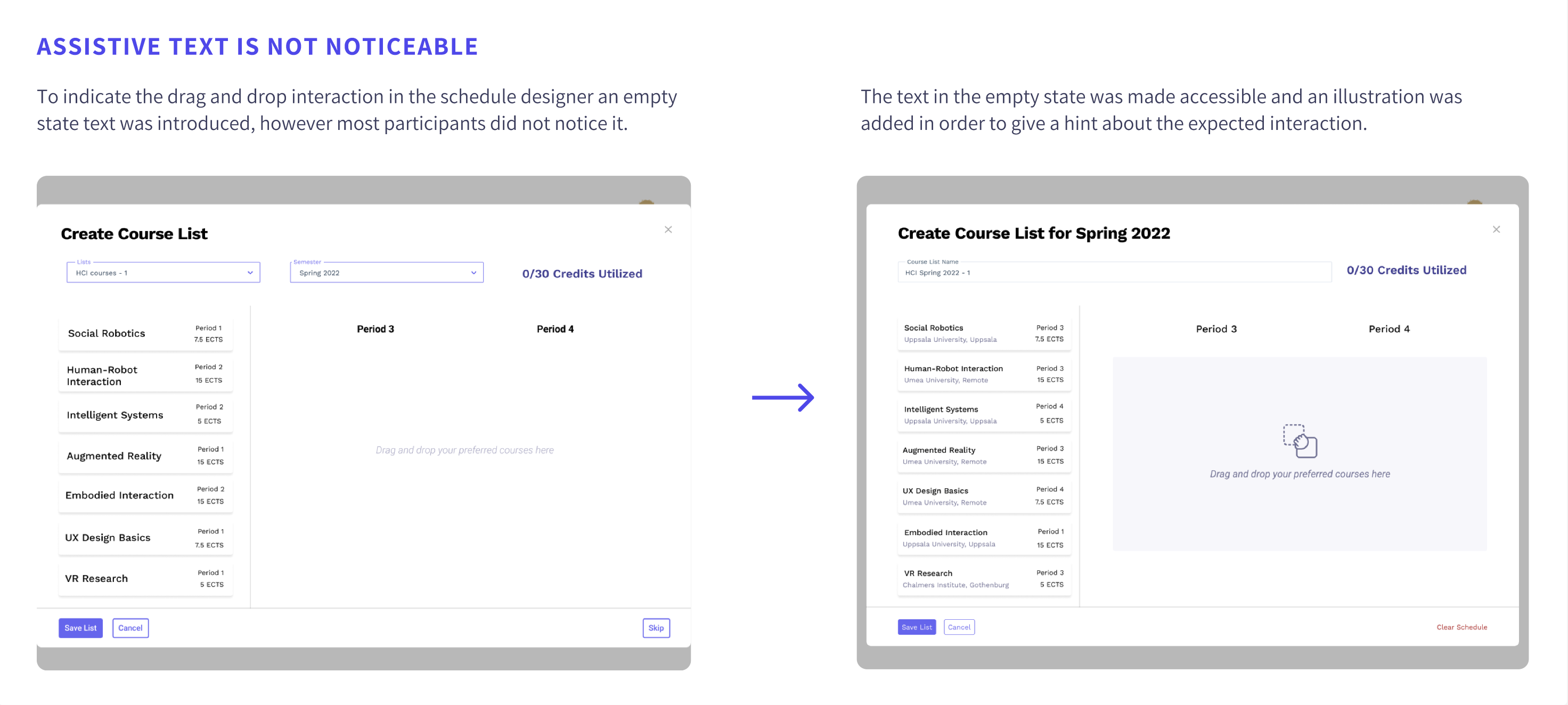
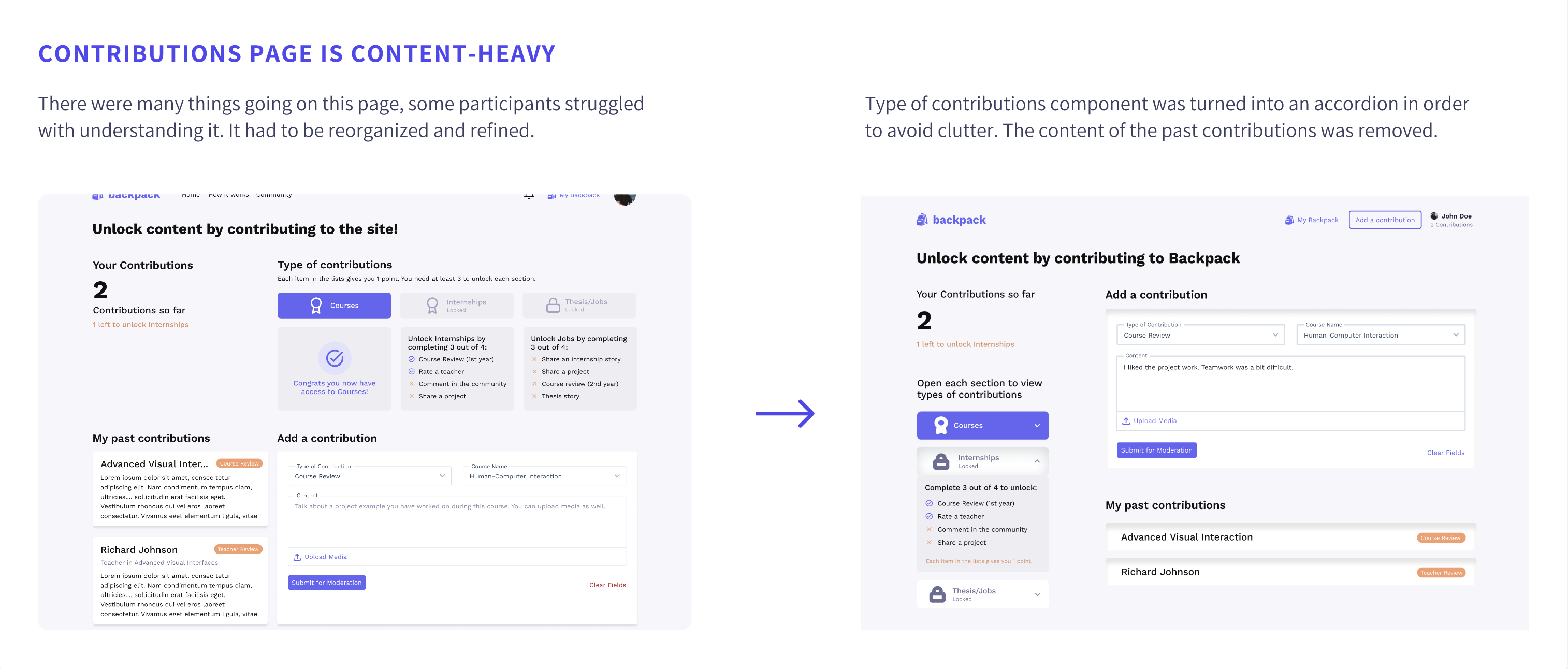
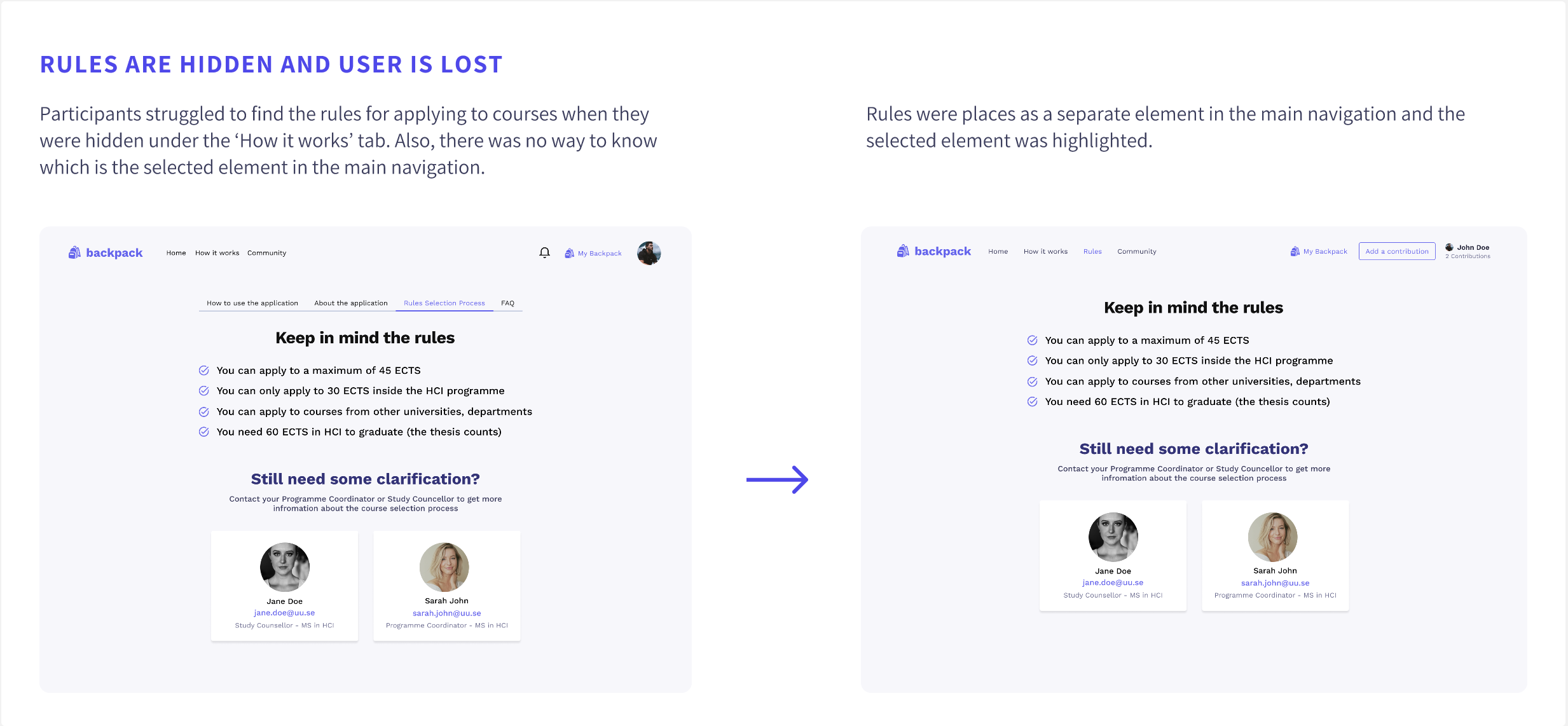